Introduction to Custom AI Applications
In the age of digital transformation, businesses are increasingly turning to custom AI applications to gain a competitive edge. These tailored solutions go beyond off-the-shelf AI tools by offering personalized capabilities that address unique organizational challenges and objectives.
Unlike generic AI tools, custom AI applications are designed to align with specific business models, industry compliance requirements, and internal workflows. They empower organizations to automate repetitive tasks, extract actionable insights from data, and enhance customer experience—all while ensuring scalability.
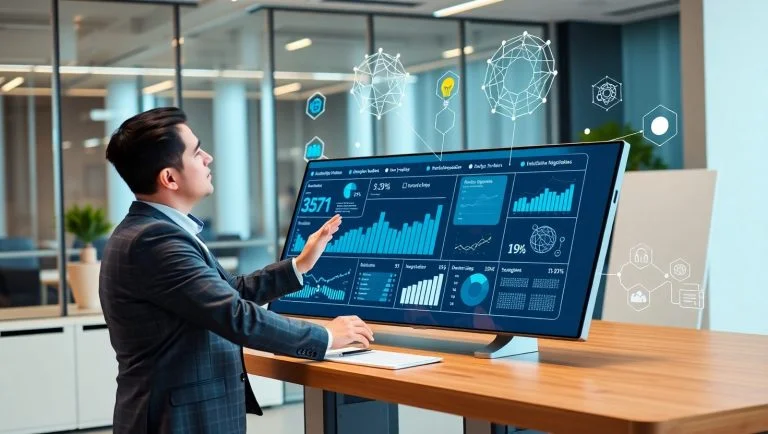
Benefits of AI in Streamlining Business Processes
Custom AI applications significantly improve operational efficiency by automating and optimizing core processes. Key benefits include:
1. Enhanced Decision-Making
AI enables real-time analytics and predictive modeling, allowing leaders to make informed decisions faster. For instance, AI can forecast inventory needs, detect fraud, or recommend financial actions based on historical data.
2. Workflow Automation
From automating HR processes to streamlining supply chains, AI reduces manual efforts and human error. AI-powered RPA (Robotic Process Automation) is being widely adopted across sectors.
3. Personalized Customer Experiences
AI algorithms help create hyper-personalized user journeys across digital channels, increasing conversion rates and brand loyalty.
4. Cost Optimization
By reducing the time and resources required for operations, AI delivers significant ROI across departments like marketing, finance, customer service, and logistics.
5. 24/7 Support
With AI chatbots and virtual assistants, businesses offer uninterrupted support, enhancing customer satisfaction and retention.
Case Studies of Successful AI Implementations
🏢 Case Study 1: Vogue Business
Challenge: Managing high volumes of unstructured customer data and digital content.
Solution: Vogue Business implemented a custom AI content curation engine that scans trends, articles, and social behavior to generate data-driven editorial suggestions.
Results: A 40% increase in audience engagement and a 30% reduction in editorial planning time.
🏦 Case Study 2: SmythOS – AI Integration in SaaS Operations
Challenge: High costs and delays in technical customer support.
Solution: SmythOS used a custom NLP-based AI chatbot to handle tier-1 support and route complex queries intelligently.
Results: 50% reduction in ticket resolution time and 70% drop in support-related expenses.
🏪 Case Study 3: Aeon Retail Chain – AI in Inventory Management
Challenge: Overstocking and understocking due to inaccurate demand forecasting.
Solution: Aeon implemented a machine learning-based demand forecasting system integrated with POS and supply chain platforms.
Results: 25% improvement in inventory turnover and 20% reduction in stock-out incidents.
💼 Case Study 4: CustomerThink – AI-Powered Customer Analytics
Challenge: Inability to map customer behavior across multiple channels.
Solution: Developed a custom AI analytics dashboard powered by sentiment analysis and journey mapping.
Results: Improved retention by 35% through targeted campaigns and optimized touchpoints.
Steps to Develop and Integrate Custom AI Solutions
Developing a custom AI application requires a strategic approach that aligns AI capabilities with business objectives. Here’s a breakdown:
Step 1: Identify Use Cases
Begin by mapping current pain points, inefficiencies, or areas where automation can deliver high impact.
Example Use Cases:
Step 2: Data Assessment and Preparation
Custom AI systems rely heavily on clean, relevant, and structured data. Assess current data quality, storage systems, and access protocols.
Step 3: Choose the Right AI Models
Select algorithms based on your use case—be it NLP, image recognition, time-series forecasting, or recommendation systems.
Step 4: Design & Development
Work with AI developers to build models, train them on proprietary data, and fine-tune the algorithms to align with operational metrics.
Step 5: Integration with Business Systems
Use APIs or middleware to integrate AI with CRMs, ERPs, and other enterprise tools. Ensure compatibility with cloud/on-prem infrastructure.
Step 6: Testing and Validation
Test the AI application in a sandbox environment. Measure KPIs like accuracy, response time, user feedback, and performance under load.
Step 7: Deployment & Change Management
Deploy incrementally, starting with pilot projects. Train your team and update SOPs (Standard Operating Procedures) to include the new AI workflows.
Step 8: Monitor, Iterate, and Scale
Post-launch, monitor model performance, retrain when needed, and scale across departments or business units.
Future Outlook of AI in Business
The future of business operations lies in intelligent automation and decision augmentation. Key trends shaping the custom AI space include:
As businesses mature digitally, AI will no longer be a luxury but a strategic imperative. Companies investing in custom AI today are setting the foundation for exponential growth, operational resilience, and sustained innovation.