What Is Generative AI?
Let’s take a quick mental detour. Imagine a painter who learns by studying thousands of works of art, eventually creating original masterpieces that capture similar moods, styles, and themes—but with a distinct twist. Now replace the paintbrush with data, and the artist with an algorithm. Welcome to the world of Generative AI.
At its core, generative AI is a branch of artificial intelligence focused on creating—rather than simply analyzing or processing—new content. This could include anything from writing emails and generating product descriptions to designing logos, creating software code, or even composing music.
The most famous examples? Think ChatGPT, DALL·E, and GitHub Copilot—all powered by large language models (LLMs) or foundation models. These models can mimic human-like behavior at scale, producing fresh and often highly convincing content across formats.
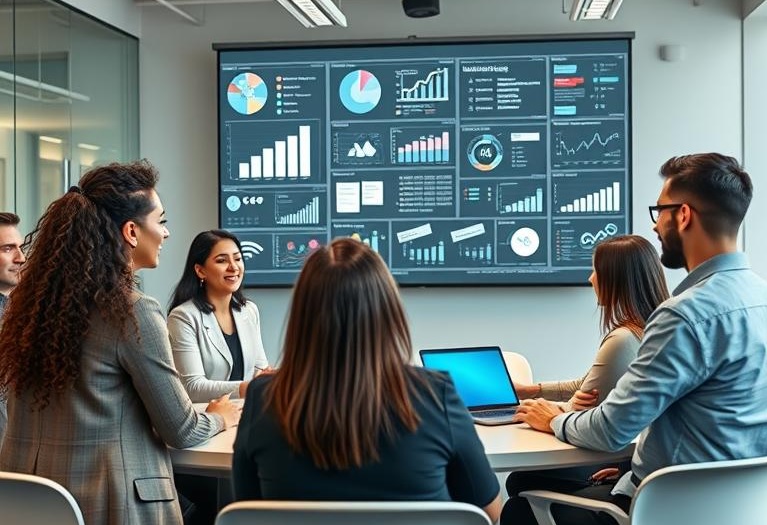
A Simple Breakdown of How It Works
If you’re a business leader, you don’t need to dive deep into neural networks to get the big picture. Here’s a simplified breakdown of how generative AI works:
- Training on Large Datasets: Foundation models like GPT-4 are trained on massive amounts of data—text, images, audio, and more. Think: books, websites, Wikipedia, product reviews, and social media.
- Pattern Recognition: The AI identifies patterns, relationships, and structures in the data. It doesn’t understand meaning like humans do, but it predicts what comes next based on probabilities.
- Content Generation: Once trained, the model can generate new content by predicting sequences—whether it’s the next word in a sentence, the next line of code, or pixels in an image.
- User Prompting: You, the user, give it a prompt. The AI processes this input and returns a generated output that matches the style and tone it learned during training.
It’s not magic. It’s advanced mathematics, paired with mind-blowing computational power.
How It Differs from Traditional AI
It’s easy to confuse generative AI with traditional AI, but they serve different purposes.
Traditional AI | Generative AI |
Primarily used for predictions and classifications | Focused on creating new content |
Examples: Fraud detection, sentiment analysis, recommendation engines | Examples: Content creation, design mockups, personalized marketing |
Operates on structured data (e.g., numbers, categories) | Trained on unstructured data (e.g., text, images, audio) |
Rule-based or supervised learning | Unsupervised or self-supervised learning |
Analogy: Traditional AI is like a calculator—it helps you analyze. Generative AI is like a creative assistant—it helps you imagine and build.
Popular Use Cases in Business
So how does this futuristic tech translate into real-world value? Here are some use cases already transforming industries:
- Marketing and Content Creation
- Customer Support
- Product Design & Prototyping
- Code Assistance
- Training & Documentation
Generate onboarding manuals, help docs, and internal FAQs tailored to your company’s tone and domain.
- Financial & Legal Drafting
- Healthcare and Pharma
In short, generative AI empowers business leaders to scale creativity, productivity, and personalization—simultaneously.
Risks and Limitations
Of course, it’s not all smooth sailing. Business leaders must remain alert to the risks and ethical pitfalls of generative AI.
- Data Hallucinations
Sometimes, AI generates false or misleading information with confidence. This is called a hallucination—and it’s more common than you’d expect.
- IP and Copyright Concerns
If an AI model is trained on copyrighted content, who owns the generated output? The legal frameworks are still catching up.
- Bias in Output
AI models reflect the biases in their training data. This can lead to discriminatory language or stereotypes, unintentionally baked into the generated results.
- Security and Privacy
If sensitive or proprietary information is used as input, how is it stored? Is it truly private? These are critical questions to ask when integrating generative tools.
- Over-Reliance and Deskilling
When AI does all the thinking, human creativity and critical thinking may erode over time. Balance is key.
The Future Outlook for Enterprises
Here’s the truth: Generative AI isn’t a fad—it’s a fundamental shift.
🔹 In the next 3–5 years, businesses that embed generative AI into their operations will see dramatic boosts in efficiency, personalization, and innovation.
🔹 Startups will use it to scale quickly and punch above their weight. Enterprises will use it to automate complex workflows and cut down costs.
🔹 From generating internal training material to creating synthetic data for R&D, the applications are nearly limitless.
However, success requires more than tools—it requires strategy.
What Should Business Leaders Do?
Final Thoughts
Generative AI isn’t just about automation—it’s about augmentation. It gives humans a creative co-pilot. But just like any tool, the impact depends on how wisely it’s used.
To stay competitive, business leaders must shift their mindset from “Can AI do this?” to “How can AI help me do this better?”
Generative AI is here. It’s evolving fast. And it’s not just rewriting content—it’s rewriting the rules of business.